The Important Role Learning Rate Plays in Neural Network Training
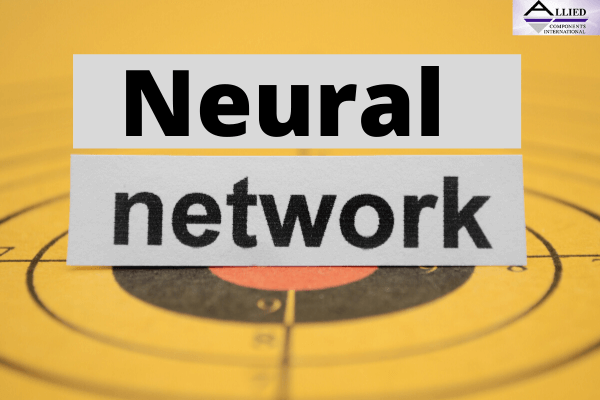
Artificial Intelligence (AI) technology is continuously expanding along with the proliferation of smart devices and deep learning, or what was originally called "neural networks." Deep learning is a subset of machine learning technology with decision-making capabilities based on historical analysis. Here's a look at how neural networks have evolved and the increasingly important role of learning rate.
Evolution of Neural Networks
The beginning of deep learning traces back to the University of Chicago in 1944. The pioneers of AI development were Warren McCullough and Walter Pitts. In 1952 they relocated to MIT as the first members of the institute's cognitive science department. Cornell University introduced the first trainable neural network called the Perceptron in 1957.
In the sixties, there was a major focus on studying these networks for neuro and computer sciences. Neural networks were designed to compute the same kind of functions as a digital computer. As early versions of machine learning, these sophisticated networks were modeled after the human brain. Computers were given training samples for analysis and for the completion of tasks.
Also Read - Silicon Photomultiplier: Everything You Need to KnowWhat is Learning Rate?
The rate at which a neural network learns is known as "learning rate." Inputting training data into the system makes it possible for the network to perform training procedures. The network weighs the data, contributing to error reduction. It's not necessary for the learning rate to be constant throughout the training process and it's possible to make it adaptive. The goal is to configure this rate using appropriate defaults with the capability of diagnosing behavior.
Also Read - Microchip Introduces Smart-Embedded Vision InitiativeDecreasing or "annealing" the learning rate is an effective strategy throughout the training. Aiming for a higher learning rate enhances the network and minimizes error, even though early network development may encompass multiple errors.
Initially, neural network technology did not survive the 1970’s computing transformation from mostly government, business and education applications to the early signs of a broader tech-filled mainstream culture craving nonstop innovation. These networks made a comeback in the 1980’s and have come and gone through many trends since then. They are steadily integrating with a diverse array of electronic components.